Understanding SVM 1000: Revolutionizing Business with Machine Learning
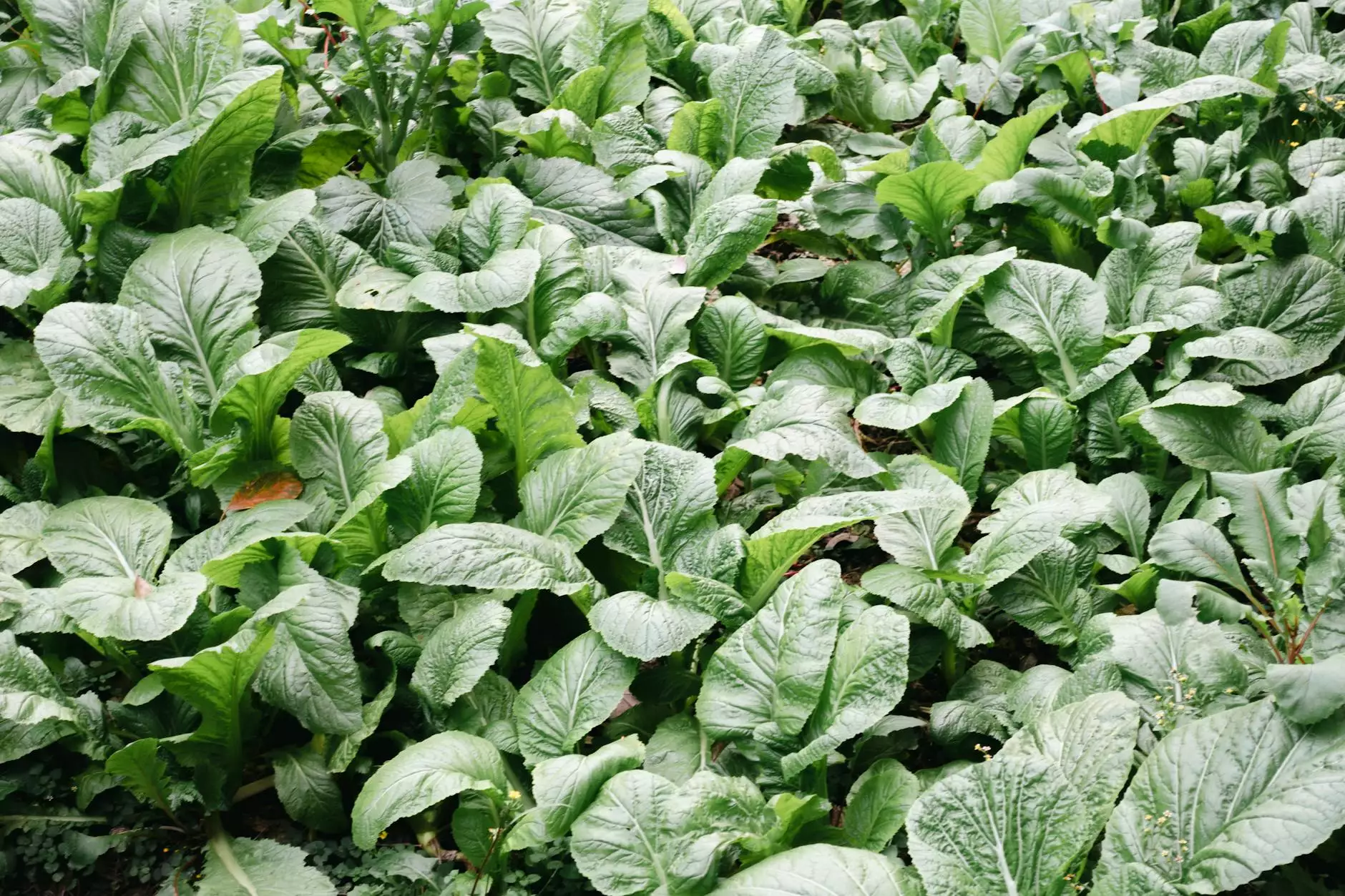
In today’s rapidly evolving digital landscape, businesses are increasingly turning to advanced technologies to gain a competitive edge. One such technology that has made significant strides is machine learning, particularly through algorithms like Support Vector Machines (SVM). In this article, we will delve into the concept of SVM 1000, exploring its implications in various business sectors, including Hotels & Travel, Hotels, and Vacation Rentals. Our goal is to provide a comprehensive understanding of how SVM 1000 can be harnessed to optimize business operations and enhance customer experiences.
What is SVM 1000?
The term SVM 1000 can be interpreted as a specific application or configuration of the Support Vector Machine algorithm, particularly when handling datasets of size 1000. Support Vector Machines are powerful supervised learning models that can perform both classification and regression tasks. They work by finding the hyperplane that best separates different classes in a dataset, thereby predicting outcomes for new data points.
How Does SVM Work?
The core principle of SVM involves identifying the optimal hyperplane that divides the classes in a high-dimensional space. To identify this hyperplane, SVM utilizes a subset of the training data known as support vectors. These support vectors are critical as they define the margin, which is the distance between the hyperplane and the closest data points from either class. The wider the margin, the better the generalization of the model. Here’s a simplified outline of how SVM operates:
- Data Preparation: Gather and preprocess a dataset, ensuring that it is cleaned and formatted appropriately.
- Feature Selection: Identify key features that significantly influence the outcome variable.
- Model Training: Use the support vector machine algorithm to train the model on the dataset.
- Hyperparameter Tuning: Optimize model parameters to enhance performance.
- Model Evaluation: Test the model on unseen data to evaluate its accuracy and effectiveness.
The Relevance of SVM 1000 in Business
The application of SVM 1000 in business cannot be overstated. Machine learning methods, particularly SVM, facilitate enhanced decision-making processes across various sectors, including hospitality, tourism, and property rentals. Below, we explore some compelling applications of SVM 1000 in these domains.
1. Enhancing Customer Experience in Hotels
Hotels can benefit immensely from employing SVM 1000 for customer segmentation and personalized marketing strategies. By analyzing customer data of size 1000 or more, hotels can accurately classify guests based on their preferences, behavior, and booking patterns. This empowers hotel managers to:
- Personalize Offers: Create tailored packages that cater to individual guest preferences.
- Optimize Pricing: Implement dynamic pricing strategies based on customer demand and market trends.
- Improve Service Delivery: Anticipate customer needs and provide proactive services that enhance satisfaction.
2. Data-Driven Decisions in Travel Agencies
Travel agencies leveraging SVM 1000 can optimize their marketing strategies by predicting customer behavior and preferences. Insights derived from historical data can help identify the target audience for specific travel packages. Key benefits include:
- Targeted Marketing Campaigns: Focus marketing efforts on specific demographics.
- Predictive Analytics: Enhance forecasts regarding travel trends based on past behaviors.
- Customer Retention: Utilize data insights to retain customers through loyalty programs and personalized communication.
3. Optimizing Vacation Rentals Management
For vacation rentals, employing SVM 1000 can streamline property management and optimize listings across various platforms. By analyzing data from past rentals, managers can improve:
- Property Pricing: Determine optimal nightly rates based on local demand and competition analysis.
- Marketing Strategies: Identify key features that attract guests to specific listings.
- Customer Feedback Analysis: Improve services and amenities based on guest reviews and satisfaction levels.
The Implementation of SVM 1000
Implementing SVM 1000 requires a strategic approach to data handling and analytical processes. Here’s a step-by-step guide organizations can follow:
1. Data Collection
Gathering relevant data is critical. Industries must not only collect quantitative data (like booking numbers and rental prices) but also qualitative data (like customer reviews and satisfaction ratings).
2. Data Preprocessing
Data must be cleaned and organized before it is suitable for analysis. Preprocessing steps may include:
- Handling Missing Values: Fill in or remove any incomplete data entries.
- Data Normalization: Scale data to ensure uniformity across different features.
- Coding Categorical Variables: Convert categorical data into a numerical format that SVM can utilize.
3. Model Training and Validation
Once the data is ready, businesses can train the SVM model using a subset of the data and validate its accuracy with another subset. Effective cross-validation techniques should be employed to avoid overfitting.
4. Continuous Improvement
Machine learning models benefit from continuous feedback. Regularly monitoring model performance and updating as new data comes in ensures ongoing accuracy and relevance.
Challenges and Considerations
While employing SVM 1000 presents many advantages, certain challenges exist that businesses should be aware of:
- Data Complexity: High-dimensional data can lead to overfitting, where the model performs well on training data but poorly on unseen data.
- Computational Resources: Training machine learning models, particularly with larger datasets, can require significant computational power and time.
- Interpretability: Understanding the predictions of SVM models can be challenging, necessitating additional tools for explainability.
Conclusion
The potential of SVM 1000 in improving business processes cannot be overlooked. From enhancing customer experiences in hotels to powering data-driven decision-making in travel agencies and vacation rentals, the application of Support Vector Machines is an influential force in today’s digital economy. By understanding and utilizing this technology, businesses can harness its power to stay ahead of competitors and meet the ever-evolving needs of customers.
As the landscape continues to change, embracing advanced machine learning strategies such as SVM will be critical for businesses aiming to thrive in competitive industries.