Mastering Data Labeling for Machine Learning: A Comprehensive Guide
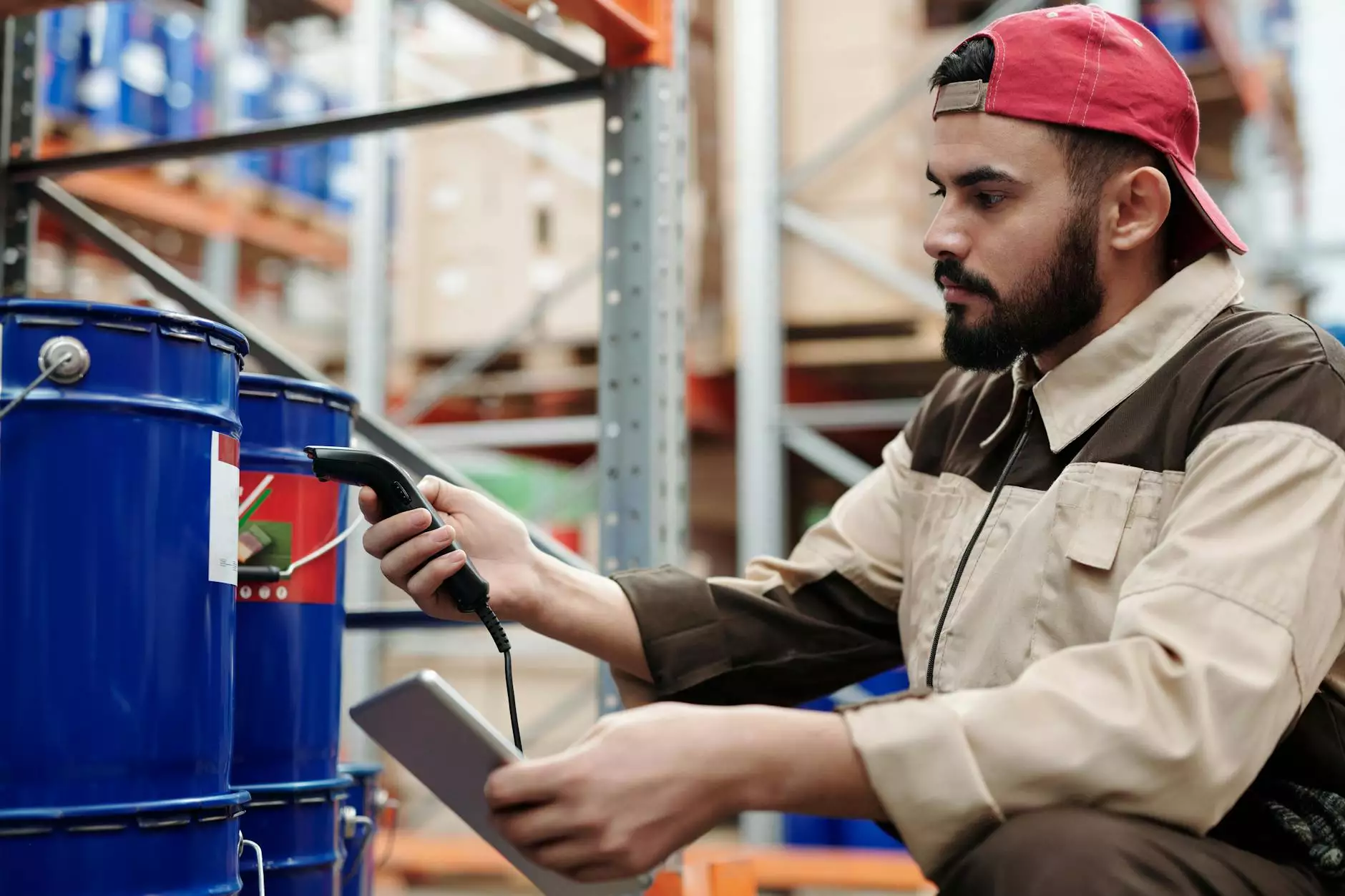
In the era of artificial intelligence, the crucial role of data labeling for machine learning cannot be overstated. As organizations seek to harness the power of machine learning, the demand for accurate and efficient data annotation is at an all-time high. This article explores the significance of data labeling, the tools available, and how businesses can leverage data annotation platforms to achieve their goals.
Understanding Data Labeling in Machine Learning
At its core, data labeling is the process of tagging or categorizing data to train machine learning models. These labels help sorting algorithms determine the features of the dataset, facilitating improved decision-making. Without proper labeling, machine learning models are prone to inaccurate outcomes, which can significantly hinder business operations.
Why Data Labeling is Essential
Data labeling enhances the performance of machine learning models. Here are some reasons why:
- Model Accuracy: Properly labeled data leads to higher accuracy in predictions, allowing organizations to make better decisions based on data-driven insights.
- Efficiency: High-quality labeled data accelerates the learning process of models, reducing the time taken to achieve optimal performance.
- Scalability: As businesses grow, the volume of data increases. A reliable data labeling strategy allows for scalable solutions that can handle large datasets efficiently.
- Versatility: Different domains require varied labeling tactics. From images to text and audio, understanding how to label different types of data is essential for comprehensive model training.
Key Components of Data Annotation Tools
To effectively manage data labeling, businesses need to utilize robust data annotation tools. Below are key components that enhance the data labeling experience:
1. User-Friendly Interface
A straightforward user interface allows for seamless interaction with the tool. Intuitive designs reduce the learning curve for new users, allowing teams to start labeling quickly.
2. Automation Features
Modern data annotation tools incorporate automation to expedite the labeling process. Automation can significantly reduce manual efforts and the potential for human error, allowing teams to focus on more complex tasks.
3. Customization Options
Organizations require different labeling approaches based on their specific needs. A versatile annotation tool should offer custom templates, allowing teams to tailor their workflows effectively.
4. Collaboration Tools
Data labeling often involves multiple stakeholders. Tools that facilitate collaboration enhance communication among team members, ensuring consistent labeling practices across the board.
5. Quality Control Mechanisms
To maintain high-quality data, annotation tools should integrate quality control features. This can include peer reviews, validation processes, and performance metrics to track ongoing improvements.
KeyLabs.ai: Leading the Data Annotation Revolution
One notable player in the data labeling landscape is KeyLabs.ai. Their innovative data annotation platform stands out for several reasons:
Advanced AI Integration
KeyLabs.ai employs cutting-edge AI technologies to streamline the data labeling process. By leveraging machine learning, their tools can automatically suggest labels, enhancing productivity while maintaining accuracy.
Robust Data Annotation Types
The platform supports various data types, including:
- Image Annotation: Perfect for computer vision tasks, including bounding boxes, segmentation, and classification.
- Text Annotation: Supports named entity recognition, sentiment analysis, and more.
- Audio Annotation: Ideal for transcribing and tagging audio files for speech recognition models.
Exceptional Customer Support
KeyLabs.ai prioritizes client satisfaction through its dedicated customer support team, ensuring users receive necessary assistance and guidance throughout their annotation journey.
Best Practices for Efficient Data Labeling
Implementing effective data labeling strategies is essential for achieving optimal results. Here are some best practices that can propel your machine learning projects forward:
1. Define Clear Guidelines
Establishing clear labeling guidelines is crucial. These guidelines should outline label definitions, annotation procedures, and specific formats to ensure consistent labeling across various contributors.
2. Train Your Team
Regular training sessions for your data labeling team can yield significant benefits. Providing updates on the latest tools, procedures, and industry standards can help improve overall accuracy and efficiency.
3. Leverage Technology
Incorporating specialized software like KeyLabs.ai's annotation platform allows businesses to automate and refine their labeling processes, significantly speeding up project timelines.
4. Review and Revise Regularly
Conducting periodic reviews of labeled data can help identify discrepancies and areas for improvement. This ensures that the data remains accurate and relevant, thus enhancing model performance.
5. Invest in Quality Control
Implement stringent quality control measures throughout the labeling process. Ensure that multiple reviewers are involved to maintain high standards of accuracy and reliability in the labeled data.
Future Trends in Data Labeling
The landscape of data labeling for machine learning is continuously evolving. Here are some trends that we can expect to shape the future:
The Rise of Automated Labeling
As AI technology advances, we anticipate an increasing reliance on automated data labeling solutions. Such technologies can drastically reduce the time and effort needed for data annotation while enhancing accuracy.
AI-Assisted Labeling Tools
We foresee innovations that incorporate AI to offer suggestions and streamline workflows in annotation tools. Such tools will revolutionize how data is processed and labeled, further improving operational efficiency.
Focus on Ethical Data Use
As data privacy concerns escalate, businesses will need to be vigilant about ethical data practices. Advocating for responsible data usage will not only benefit organizations but enhance their reputations in the marketplace.
Collaboration Across Industries
An increasing number of companies will seek partnerships with data labeling specialists to improve their machine learning projects. This trend will accommodate diverse industries that require high-quality labeled datasets.
Conclusion
In conclusion, data labeling for machine learning is an essential component of any AI-driven project. By understanding its importance and utilizing the right tools such as those offered by KeyLabs.ai, organizations can significantly enhance their data annotation processes. Implementing best practices and staying aware of future trends will ensure companies remain competitive in the dynamic AI landscape.
Embrace the future of machine learning with effective data labeling — the key to unlocking the full potential of your data.
data labeling machine learning